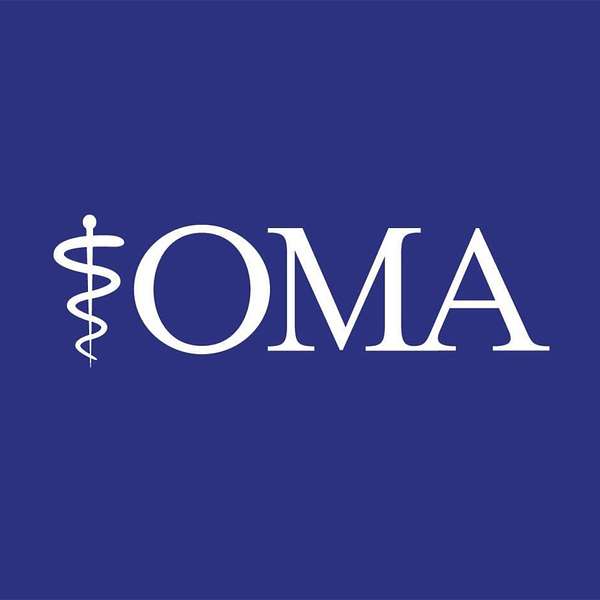
OMA Spotlight on Health
OMA Spotlight on Health
Paving the way with medical technology
Ontario physicians are amid a digital transformation, adopting increasingly sophisticated technologies that help better serve patients and simplify day-to-day operations across health-care settings. In the first episode of this two-part podcast, four physicians – Dr. Chandi Chandrasena, family physician in Ottawa and chief medical officer at OntarioMD; Dr. Amol Verma, physician of general internal medicine at St. Michael’s Hospital and co-lead for Gemini (hospital data and analytics study); Dr. Teodor Grantcharov, professor of surgery at the University of Toronto and keenan chair at St. Michael’s Hospital; and Dr. Muhammad Mamdani, vice president of data science and advanced analytics at Unity Health Toronto – discuss the development and use of medical technology within Ontario’s health-care system. From electronic health records, wearables and medical apps to artificial intelligence tools and data analytics and standardization, these diverse tools are changing how we manage, monitor and evolve our health-care system.
(Background music begins)
Georgia Balogiannis: In this podcast the Ontario Medical Association looks at current issues of interest in health care. Spotlight on Health gives you all the straight talk. We're Ontario's doctors and your health matters to us. I'm Georgia Balogiannis for the Ontario Medical Association.
The pandemic necessitated a pivot to virtual medical appointments for many patients, and electronic health records, medical apps, and digital data have become the norm in Ontario's healthcare system. In part one of a two-part episode, physicians discuss the developing role of medical technology. Dr. Chandi Chandrasena is a family physician in Ottawa and chief medical officer at OntarioMD.
(Background music swells and fades)
Dr. Chandi Chandrasena: First, I want to say that virtual care is still care, and digital health is still health. So, it's not different than any of the care that physicians have been providing for their patients, or you know, what we do on a daily basis, it's just a different way of providing that care that we've always done.
And so, with COVID, I think there was a bit of a rocket fuel that happened, where it just made virtual care take off. And so now we're kind of looking at it going, “okay, is this the best modality for everything?” because of the pendulum kind of swung towards virtual care only as opposed to in person care, and now it's kind of coming back and equalizing a bit, and we're just trying to figure out, what's the right balance?
You don't necessarily have to come back into my office anymore. We can do that over a secure platform. So, it really allows for you to have the convenience of staying home and it allows me to still contribute to your care without having to bring you in, and technology does that. It also allows me to send you messages that I want to send you about your health, and it allows that back-and-forth, but doesn't necessarily mean you have to book an appointment and come in and see me. So, I'm a lot more accessible to you for when you need me.
When we introduce technology into anything, we always have to consider how is it going to be used by the end user. So, in this case, it's physicians and really the end users are the patients. So, if for some reason physicians aren't able to use it, it's not going to work no matter how phenomenal we think the technology is going to be. And, by that same token, if it's not really going to be used by the patients, again, it doesn't matter how phenomenal we think it's going to be, it's just not going to be practical and useful.
I think when we look at technology, we have to look at the pros and cons of it, especially when we're delivering care virtually. So, there's a lot of pros, because now we can do it asynchronously — you don't have to come and fight traffic, you don't have to come into my office. It allows me that flexibility where I don't need to be in an office from nine to five, let's say, and it also allows me the flexibility for my work/life balance in general.
But this care also has negatives. And I talked a little bit about that ‘end user’ piece in that it has to be a tool that's easy to use, integrated, and that patients can use. And that's really where sometimes we don't think this through because we have a one size fits all. And we're just early stages, but now we have to start thinking of, okay, what works for certain populations? We have to be careful that when we use these technical tools that we're not creating an equity issue because then we start separating patients that understand tech — can get on it seamlessly, can use their iPhone or their smartphone, can use their iPad and they can do it very simply, and they can access the system — which means that I'm more accessible to them, as opposed to patients who don't have that luxury —maybe they don't understand how to use it that well, they don't have iPads, iPhones, smartphones, the technology itself, they don't have good internet — so now I'm not so accessible anymore.
There are a lot of conditions that just cannot be assessed virtually and you just have to come in. And so, if you're just a clinic that's only available virtually and there's no means for me to actually see you, then it's just not providing the care. You could miss a lot of diagnoses that way.
(Background music swells and fades)
Dr. Chandrasena: There's two things that we're working on in health care right now, and one is getting that population health data together and that interoperability of data and that's where these wearables also fit in. The other part is the validity of the data. So, not all wearables are created equally; they're not necessarily validated.
There's a number of times that my patients have come in and said, "Oh, I think my heart rate is really irregular because my watch is telling — look, it's happening again." But when I actually check them it's perfectly fine, it's just that there's a glitch or their app isn't registering it. We can't take all data as being equal, and dumping it into a repository where that data is validated the same as other data. And that creates a bit of a problem. So, not only are the platforms different, so they don't talk to each other, but the data that's coming isn't necessarily validated.
And then the other part is privacy and security, right? We have to be really careful about what we're sharing data-wise, who has access to that data. And, again, apps are not created equal. Some apps will share your data with a lot of third parties. And I always do this medical apps talk for patients, and I always warn them that they have to look at the sharing and the privacy and the validity and what it's meant for. And a lot of these apps that patients take as being medical apps are not regulated. They're just meant to be for, in quotes, "fun." So, if it's in quotes "fun," then we're not really sure how valid it is.
There's a wonderful sensor that I use on my patients, and they're able to download it. They're not able to send it to me digitally because it doesn't incorporate with my system, and I would love for that to happen in the future. It's just not available right now. And if we were going to incorporate it into the system, it would have to be a seamless workflow. Because again, I don't want to be spending 5-10 minutes with every patient who has an iWatch to download their data, put it into my system, have a look at it — that time can be used more efficiently in a better manner. So, that integration piece just isn't there, and I think we have to work on that.
(Background music swells and fades)
Balogiannis: Dr. Amol Verma is a general internist and health services researcher at St. Michael's Hospital and an assistant professor at the University of Toronto, as well as the co-lead for GEMINI, a hospital data and analytics network.
(Background music swells and fades)
Dr. Amol Verma: My research really started with a core question which is, “what is the quality of care delivered on general internal medicine units?” And so, we started working with colleagues across hospitals, in the Toronto area initially, to extract information from hospital electronic records about all of the details of about inpatient care. So, things like what rooms a patient stayed in, what clinicians took care of that patient, what kinds of tests and treatments were ordered, from medications to interventional procedures, we got information about diagnostic imaging. We collected all of that information and we brought it together in a single database so that we could start asking and answering questions about the quality of care that's being delivered on general internal medicine units.
And the reason this is really important is that general internal medicine units have seen a large growth in the volume of patients over time. And we know that, as our population ages and with a growing prevalence of chronic illness, many people now have multiple coexisting conditions. And so, many more people are being hospitalized on a general medicine unit because, not only did they have a heart problem that brought them to hospital, but they also have kidney problems or liver problems.
And so, this new generalist inpatient model of care is becoming increasingly prevalent, and unfortunately most research and quality improvement activities have historically been focused on single disease entities. And so, my research was really focused on understanding that general medicine care for people with multiple coexisting conditions.
And we started by going to where the data was available, and that was from computer information systems at hospitals. And as a result, we've now grown and become one of Canada's largest digital health platforms for research, where we now extract data from more than 30 hospitals all across the province who care for more than 60 percent of the province’s hospitalized adult patients.
One of the most important challenges when it comes to drawing insights from routinely generated data in the health system is the fact that those data are highly unstandardized across different institutions. And so, every organization has its own way of dealing with data — it has its own naming conventions, its own approaches to ensuring data quality. And so, when you try to aggregate or centralize data, you find that it's very much a dog's breakfast. There might be 15 different names for a single lab test just across two or three organizations. And so, trying to standardize and harmonize all of that is a big part of the work that we do at GEMINI.
There are lots of insights that have been generated from these data essentially showing clinicians opportunities to improve care in comparison with their colleagues, whether that be related to the way that they order diagnostic imaging or bloodwork or blood transfusions, or thinking about higher-level indicators, things like hospital readmission, or hospital length of stay.
I would say the second big area of insight that we've identified has been lots of opportunities to improve the way we use certain resources in hospitals. There are a lot of detailed and specific insights that we've generated and now we're really working on continuing to generate those insights, but also translate some of those findings into practice change.
(Background music swells and fades)
Dr. Verma: Typical clinical practice will undoubtedly be touched by this data revolution. And the average doctor of tomorrow, or frankly of today, needs to be data savvy in a way that we didn't need to be a decade ago. They need to be able to access and easily use numerous digital systems but, also, they need to be able to think about data and analytics.
They’re, people are increasingly receiving their own practice data and feedback, but they also are receiving increasing decision support from tools, whether that be artificial intelligence tools or otherwise that are embedded in their electronic medical record. So, I think clinicians need to become more sophisticated consumers and users of these tools.
It's actually quite analogous to some of the other technologies in medicine. So, I have to be able to understand how an MRI machine is used. I do not need to know how the actual machine works. I don't know anything about photons and spin and whatever is happening inside of that big magnet, but I know how to use the technology. I know when it should be used, I know when it should not be used, and I know how to interpret its results and its limitations. And I think it's reasonable to apply that same lens to thinking about data, artificial intelligence, and all of these other new digital solutions.
I'd like to tell you about two interesting applications of artificial intelligence in healthcare that I've been a part of and that are really exciting to me. All of my work in artificial intelligence in medicine is oriented around clinical problems. And so, the first clinical problem is the problem of delirium.
Delirium is a state of acute confusion that affects 20 to 30 percent of hospitalized adults. When adults are affected by delirium in hospital, they have much worse health outcomes. My team has developed an artificial intelligence solution that takes all of the information in an electronic medical record and can accurately identify patients with delirium, and also predict the risk of developing delirium in the future.
A second clinical problem that is ripe for intervention with artificial intelligence is the problem of patient deterioration in hospitals. One of the hardest things for clinicians to predict is which patients are going to deteriorate. This is an opportunity for an artificial intelligence tool. So, at St. Michael's Hospital, we created a tool called CHARTWatch, which takes about 100 inputs from the hospital's electronic medical record and produces an hourly prediction of the patients who are at highest risk of deteriorating in the future.
One of the things we found with that tool is that the combination of the tool prediction and clinical judgment is 15 percent more accurate in predicting patient deterioration than clinician judgment alone. So, it's really showing there's value there and opportunity to improve the way we care for patients.
(Background music swells and fades)
Balogiannis: Dr. Teodor Grantcharov is a professor of surgery at the University of Toronto and Keenan chair of surgery at St. Michael's Hospital.
(Background music swells and fades)
Dr. Teodor Grantcharov: How did the whole story about the black box start? I was a resident in Copenhagen, and that was early 2000s and I was doing my PhD. And the focus of my PhD was designing a virtual reality system to measure the skill of the operating surgeon.
At that point, early 2000s, that wasn't really a big thing. We all assumed that once you become a surgeon, you're good enough, and there is very little variability in our skill. But what we found out with this measurement system that we designed in Copenhagen, was that there was a huge variability in our performance, which kind of surprised us a little bit. We found that there was a huge variability between individuals but also a huge variability within the same individual depending on whether they were tired, were distracted, and so on.
At that point, also, there was emerging evidence that there was a significant variation in clinical outcomes. So, we wanted to find this link between performance and clinical outcomes. The problem was that we could do it in a virtual environment, we never had access to data from the operating room. The operating room was a black box that nobody understood.
That's where everything started. That's where I got the idea that, probably, if we find a way to bring more transparency in the operating room and capture more high-quality data, we will be able to understand the factors that influence our performance. And then also how our performance in real environment, in real clinical environment, correlates with clinical outcomes.
So, we found a massive amount of obstacles — there were technological obstacles, cultural obstacles. When the surgeon-in-chief at St. Michael's Hospital offered me the job at St. Mike's, that was one of the promises — that we will have the opportunity to build this system at some point in Canada, and it did become a reality in 2014.
The first generation of the black box, which was introduced there in 2014-2015, was very hardware heavy. So, you could see a little device that looked like a DVD recorder, and there are a few cameras and microphones in the operating room. We replaced that with second generation, which is very hard to see. It is all based on software — it has a lot of integration to devices, information systems in the hospital; it does still acquire audio-visual data. What we see is the final outcome, the output of the black box, which is deep analytics on things that matter — things like performance, efficiency, compliance to standard operating procedures — and also it gives us the opportunity to utilize the power of video to improve performance and coach each other.
(Background music swells and fades)
Dr. Grantcharov: The common question: do we see improvements as a result of this intervention? And yes, we have. We see it every day, and we've studied many of them. A lot of the improvements in other high-risk industries — like aviation, oil, nuclear, military — have been a result of this type of data and analytics. So, certainly the concept is not new. It is new in health care. And we keep seeing new success stories every day, particularly around improving performance and reducing adverse events and adverse outcomes.
We hope in the coming years to see much more significant and widespread growth in adoption. We recently signed an agreement, also, with Queen's University in Kingston to use it to measure and improve performance for residents in general surgery.
Traditionally, and still the natural reaction when you see this technology in the operating room, is anxiety. We are used to working in a very un-transparent and secretive environment, and suddenly we bring a lot of light — we illuminate the black box of surgery. Anxiety is a natural first reaction, but once doctors, nurses, and other staff that benefit from this information, start seeing the results, start seeing the analytics, they understand the power. And certainly, we've seen this transition from individuals who are resistant or anxious to the same individuals becoming some of the biggest supporters. Because we're all competitive people. We want the best for our patients. We, all of us, wake up in the morning and we want today to be better than yesterday and tomorrow to be better than today. And without this type of information, without data about what we do right and what we do wrong, it's very difficult to improve.
A lot of the findings, a lot of the information, the processes we use to extract information, are very transferable and provide value to any surgical procedure. We've demonstrated consistently that the success of our work is not possible without the team. We currently provide a lot of information to allow other professional groups like nursing, respiratory therapists, including the — also —the cleaning crews in the operating room. The entire team need to get access to this information, need to get opportunities to continuously improve. Not only improve their performance, but also improve teamwork, communication, collaboration and, in general, support each other in order to provide the best possible care for our patients.
The patient is the most important stakeholder in the operating room. Helping them understand what happens there — and probably not to the most critical detail, but in general – of how we make decisions, how we improve, and what the processes we've introduced to make sure that they will be safe in the most vulnerable moment of their lives, is critical.
Whenever I can send patients for surgery or share with them the concept of the black box and share with them how we use it, the response is always the same. I will say that probably more than 90 percent of the patients respond to that with the comment, ‘I can't believe this hasn't been done before in healthcare. I always assumed that there was a system in place that helped you learn from your errors and continuously improve.’
Patients don't expect zero errors, but they expect a system that makes sure that we learn from our errors and make it much more difficult in the future to repeat them.
(Background music swells and fades)
Balogiannis: Dr. Muhammad Mamdani is the vice president of data science and advanced analytics at Unity Health Toronto, and the director of the University of Toronto Temerty Faculty of Medicine Centre for Artificial Intelligence, Research and Education.
(Background music swells and fades)
Dr. Muhammad Mamdani: The use of artificial intelligence in the day-to-day functioning of a hospital can be highly, highly variable, because there are many, many problems that can be solved with artificial intelligence or a machine-learning algorithms.
Nurse staffing is incredibly challenging in hospitals across the country. How do we optimize our staffing? We have teams called nurse resource teams. And these are teams of nurses that actually relieve nurses that take leave, whether they call in sick or go on vacation or whatever have you. Well, we can optimize the size of these nurse resource teams using machine learning, using the optimization algorithms, to tell us you know, actually, you should be shooting for ‘X’ because that's going to minimize costs and maximize the number of nurses that you need for efficient care to get you to deliver care that is optimal for patients.
Perhaps the third example I can give is how we actually automate information extraction. We have a tool we affectionately call ‘muskrat’ in our multiple sclerosis clinic, where it is able to, quote unquote, "read" six, seven years of history around a patient within seconds and summarize the key points for our clinicians, so they're not really digging through those notes and spending hours trying to find valuable information.
We've developed and deployed over 40 of these solutions into clinical practice. So, we've kind of had some war wounds, and learned a few things the hard way. But our model is fundamentally different from a lot of places. Our team of data scientists — we actually have a team of about 30 data scientists — and our general rule is that the questions and the issues should not be coming from the data science team — they should be coming from the end users. They have to be the champions, back on the floors, and say, you know, look, I'm developing this with this team, we as a community have a big problem — we can solve this problem, but we have to do it together.
(Background music swells and fades)
Dr. Mamdani: The issue of data literacy is incredibly important. Do patients understand the importance of this data, how it may be used to help provide better care? Do clinicians understand the importance of this data and how it can be used to drive patient care?
We haven't leveraged our data nearly as effectively as some of the other sectors. We have Amazon that's transformed the commerce side, and how Google Maps can usually pretty accurately get us to where we want to go most efficiently. And yet, in healthcare, we're still fumbling with our fax machines.
I would say us understanding the power of this data, to value that data, to use that data in a way that can drive more effective care, we need to still do a lot of work on. No longer should it be that “my data are my data because I don't trust anybody, and I'm not going to let anyone use it to advance care.” But it should be an understanding of, “oh, this is what you're going to do with my data — it means not only better care for me, but societal benefits. I want the right safeguards, absolutely, but I want my data to contribute to better health for everybody.” That's the understanding that I think we're driving towards.
We need to enable the infrastructure and the technology. And yes, we will work out issues around privacy, we will work out issues with us coordinating better and engaging people better. I think we could really transform medicine — not change medicine — I think we could transform medicine into something that is just so much more effective and more powerful for our patients.
(Background music swells)
Balogiannis: This podcast is brought to you by the Ontario Medical Association and is edited and produced by Jodi Crawford Productions. To learn more about the Ontario Medical Association, please visit oma.org.
(Background music fades)